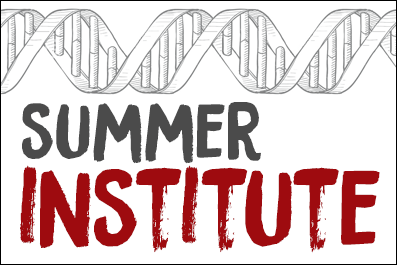
UCLA Computational Medicine Faculty Positions
The UCLA Department of Computational Medicine is looking to hire new faculty. Please pass this advertisement to anyone who may be interested.
The David Geffen School of Medicine (DGSOM) is searching for tenure-track faculty to join the Department of Computational Medicine. The successful candidate will enjoy a richly collaborative working environment and a possible joint appointment with the UCLA Henry Samueli School of Engineering and Applied Sciences (HSSEAS), the UCLA Division of Life Sciences, or the Departments of Biostatistics or Epidemiology in the Fielding School of Public Health (FSPH). We are seeking candidates with strong research programs in the quantitative biomedical sciences, with emphasis on the development and use of novel mathematical models and computational methods. We plan to hire at the Assistant Professor level but will certainly consider exceptional candidates at higher ranks.
The DGSOM and HSSEAS have invested in Computational Medicine to lead the transformation of biomedical sciences by leveraging recent advances in the mathematical, computational, and data sciences. The recently established Institute for Precision Health in the DGSOM provides unique opportunities to build upon successful collaborations between these two schools and encourage new ones. UCLA plans to hire multiple faculty over the next few years with diverse research interests in applications across biology, medicine, and public health. Some of the faculty will be hired to support the Department of Computational Medicine’s Biomathematics Graduate Program, a leading program nationally with a long and illustrious tradition, and these faculty will be expected to play an active role in the program. Candidates for these positions will be judged on productivity, creativity, commitment to realistic biological models, mathematical sophistication, and ability to collaborate across disciplinary boundaries. Faculty will also be hired in other interdisciplinary areas of interest including Computational Genomics, Clinical Machine Learning, and Computer Vision applied to Medical Imaging.
Candidates should hold a Ph.D., M.D., or equivalent by the date of appointment and provide the potential for scholarly impact through publications, excellence in teaching, and strong oral and written communication skills. We welcome candidates whose experience in teaching, research, or community service has prepared them to contribute to our commitment to diversity and excellence. Faculty appointment level and salary will be commensurate with the candidate’s experience and qualifications.
Applicants should submit a cover letter, curriculum vitae, list of three references, research statement, teaching statement, and statement of contributions to equity and diversity inclusion to Bogdan Pasaniuc, Chair of the Computational Medicine Faculty Search Committee.
Many of the on-campus interviews will occur during January 21-22 and February 18-19, 2020.
Questions about the search should be directed towards Bogdan Pasaniuc (pasaniuc@ucla.edu) Chair of the Search Committee or Eleazar Eskin (eeskin@cs.ucla.edu) Chair of the Department of Computational Medicine.
Apply at:
https://recruit.apo.ucla.edu/JPF04995
The University of California is an Equal Opportunity/Affirmative Action Employer. All qualified applicants will receive consideration for employment without regard to race, color, religion, sex, sexual orientation, gender identity, national origin, disability, age or protected veteran status. For the complete University of California nondiscrimination and affirmative action policy, see UC Nondiscrimination & Affirmative Action Policy.
http://policy.ucop.edu/doc/4000376/NondiscrimAffirmAct
Computational Genomics Summer Institute 2019

Computational Genomics Summer Institute – Apply Today
CGSI – extended application deadline
Rolling admissions are starting March 15th.
Registration Fee:
$550 apply by April 1st
$650 apply by April 15th
$750 apply after April 15th
Subsidized housing for CGSI is guaranteed for anyone who applied by April 15th. Housing for applicants who apply after April 15th will be given on a first come first serve basis subject to availability.
We have filled most of the slots in the 2018 CGSI Long Course, however, there are still a few available slots.
The long program has been a huge success last year and many people were not able to be admitted as they did not apply on time – make sure that this year you are not left behind!
There are also still available spaces in the 2018 CGSI Short Course.
Register now to get the lower rate and subsidized housing.
DATES
SHORT PROGRAM #1: July 16 – 20, 2018
SHORT PROGRAM #2: July 30 – August 3, 2018
LONG PROGRAM: July 11 – August 3, 2018
@ UCLA Campus, Los Angeles
Visit our website to learn more.
The application is open!
Apply now for this upcoming summer’s Short and Long Courses:
STEP #1 APPLY
STEP #2 SEND YOUR CV
Watch the best talks in 2018 CGWI
Our first offering of the Computational Genomics Winter Institute was a success. In our feedback survey, we asked the participants to pick three talks they wanted to highlight on our website. We would first like to emphasize that the feedback we got was that all the talks in CGWI were excellent. But we are happy to announce that the ones that received the most votes are the talks of Brian Browning, Casey Greene, Su-In Lee, and John Novembre. We now have links to these videos highlighted on the front page of CGWI for easy access, and links to all of the talks are also available at the CGSI website.
Su-In Lee: “Interpretable Machine Learning for Precision Medicine.”
Casey Greene: “Deep learning: privacy preserving data sharing along with some hints and tips.”
John Novembre: “Computational tools for understanding geographic structure in genetic variation data.”
The 2018 CGSI Organizers
CGSI Co-organizers:
Fereydoun Hormozdiari, UC Davis
David Koslicki, Oregon State University
Kirk Lohmueller, UCLA
Ran Blekhman, University of Minnesota
CGSI Program Co-directors:
Eleazar Eskin, UCLA
Eran Halperin, UCLA
Dima Shlyakhtenko, UCLA IPAM
CGSI Steering Committee
Eleazar Eskin, UCLA
Eran Halperin, UCLA
John Novembre, University of Chicago
Ben Raphael, Princeton University