This week, our group published a paper in the American Journal of Human Genetics that presents a new computational method for improving the accuracy of genome wide association studies. ZarLab alumni Farhad Hormozdiari (PhD, 2016) developed the method, CAVIAR (CAusal Variants Identification in Associated Regions), a statistical framework that quantifies the probability of each variant to be causal while allowing an arbitrary number of causal variants.
Genome-wide association studies (GWASs) identify genetic variants associated with diseases and traits. Recent successes in GWASs make it possible to address important questions about the genetic architecture of complex traits, such as allele frequency and effect size. A more comprehensive understanding of these aspects will guide the development of new methods for fine mapping and association mapping of complex traits—and the discovery of new biomarkers for disease diagnosis and treatment.
One lesser-known aspect of complex traits is the extent of allelic heterogeneity (AH). Allelic heterogeneity occurs when different mutations at the same locus affects the same phenotype. AH is very common in Mendelian traits, but we know little about the extent to which AH contributes to common, complex disease. Undetected AH could potentially bias results of an association study, leading to false positive results.
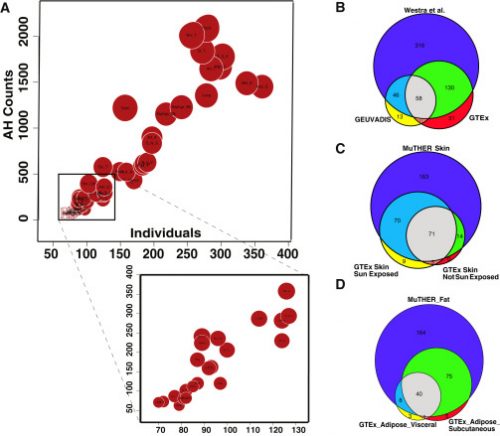
Levels of Allelic Heterogeneity in eQTL Studies. For more information, see our paper.
In order to take AH into account while conducting a GWAS, we developed a computational method to infer the probability of AH. Our method quantifies the number of independent causal variants at a locus that can be responsible for the observed association signals detected in a GWAS. Our method is incorporated into the CAVIAR approach, and it is based on the principle of jointly analyzing association signals (i.e., summary level Z-scores) and LD structure in order to estimate the number of causal variants.
Our results show that our method is more accurate than the standard conditional method (CM). We applied our novel method to three GWASs and four expression quantitative trait loci (eQTL) datasets. We identified a total of 4,152 loci with strong evidence of the presence of AH. The proportion of all loci with identified AH is 4%–23% in eQTLs, 35% in GWASs of high-density lipoprotein (HDL), and 23% in GWASs of schizophrenia. For eQTLs, we observed a strong correlation between sample size and the proportion of loci with AH, indicating that statistical power prevents identification of AH in other loci.
One of the main benefits of our method is that it requires only summary statistics. Summary statistics of a GWAS or eQTL study are widely available, so our method is applicable to most existing datasets. We have shown that AH is widespread and more common than previously estimated in complex traits, both in GWASs and eQTL studies.
Our results highlight the importance of accounting for the presence of multiple causal variants when characterizing the mechanism of genetic association in complex traits. Falling to account for AH can reduce the power to detect true causal variants and can explain the limited success of fine mapping of GWASs.
In a related study, researchers at University of California, Irvine, and University of Kansas, identified an analogous signal in eQTLs from genetic sequencing of flies. King et al. (2014) observe that the vast majority of genes with eQTL are more consistent with heterogeneity than bi-allelism. Read more about this related study, “Genetic Dissection of the Drosophila melanogaster Female Head Transcriptome Reveals Widespread Allelic Heterogeneity.”
CAVIAR was created by Farhad Hormozdiari, Emrah Kostem, Eun Yong Kang, Bogdan Pasaniuc and Eleazar Eskin. Software is freely available for download: http://genetics.cs.ucla.edu/caviar/
For more information, see our full paper, which can be accessed through AJHG: http://www.cell.com/ajhg/abstract/S0002-9297(17)30149-0
The full citation of our paper:
Hormozdiari F, Zhu A, Kichaev G, Ju CJ, Segrè AV, Joo JW, Won H, Sankararaman S, Pasaniuc B, Shifman S, Eskin E. Widespread allelic heterogeneity in complex traits. The American Journal of Human Genetics. 2017 May 4;100(5):789-802.